|
IEEE WCCI 2022 Returns to In-Person Conference
IEEE WCCI 2022, first in-person conference since COVID, has been held in 18-23 July at Padova, Italy. To improve the quality of reviews, a paper bidding feature was introduced for the first time, which was supported by the Microsoft Conference Management Toolkit (CMT) submission system.
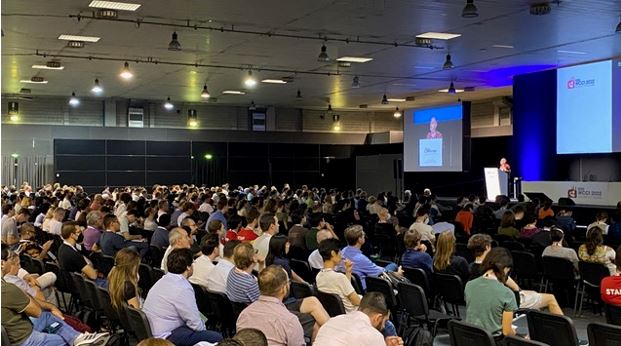
In total, 2,068 expert reviewers contributed to a rigorous double-blind peer-review process involving at least three reviewers for each of the 2,646 paper submissions received from over 73 countries across all continents. Overall, 1467 high-quality papers were accepted, resulting in a program containing 934 oral presentations organized in 255 sessions, including 87 special sessions, and 533 posters presented in 5 sessions within IJCNN. Highlights of the program were 5 plenary speeches by Jim Keller (IEEE Rosenblatt Award recipient for 2021, and President of IEEE Computational Intelligence Society for 2022-2023), Paul Werbos (IEEE Rosenblatt award recipient for 2022), Michael Bronstein, Bing Xue and Tinne Tuytelaars.
Alessandro Sperduti and Marco Gori
General Co-chairs of IEEE WCCI 2022
|
|
|
|
IEEE CIS WCI Reception at WCCI 2022
After two years of meeting virtually we were able to meet in-person once again at the IEEE CIS WCI reception at WCCI. The aim of the reception is to acknowledge and support women in Computational Intelligence. The informal nature of the event allowed for old friends to catch up and new friends to be made.
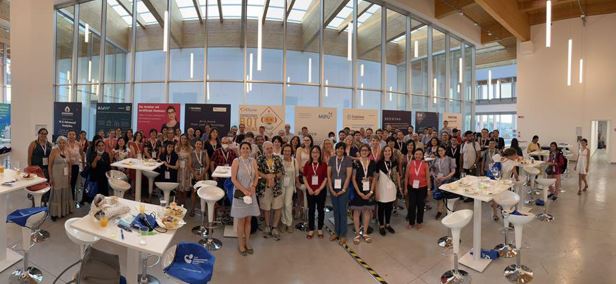
We were fortunate to have two fantastic guest speakers, both leaders in the field of computational intelligence, share their experiences with us. Alice Smith presented highlights from "Women in Computational Intelligence-Key Advances and Perspectives on Emerging Topics", a recent publication by Springer which she edited, showcasing women researchers in Computational Intelligence. Bing Xue, one of the youngest full professors in computational intelligence, shared with us lessons learnt in her journey from PhD student to full professor.
A big thank you to the IEEE CIS WCI subcommittee and Jo-Ellen Snyder for organising the event, our president Jim Keller for his welcome, both our guest speakers for their inspiring presentations and sharing their wisdom with us and the attendees for making the event such a vibrant one!
Nelishia Pillay
Chair of the IEEE CIS WCI Subcommittee
|
|
|
|
CI & AI-FML Machine Learning Competition @ IEEE WCCI 2022
CI & AI-FML Machine Learning Competition for Human and Smart Machine Co-Learning on Real-World Applications
Congratulations to the competition winners! The competition results are as follows: (1) Category A: Winner is Team Double A, First runner-up is Team Power Samurai, and Second runner-up is Team Weather Goddess. (2) Category B: Winner is Team CI Super, First runner-up is Team CI Star, and Second runner-up is Team CI Little Vanguard.
Summary Report and Result Summary Report is as follows: (1) 30 Entries Registration for Phase-1 Competition, including Sixteen teams registered for Category A, Eight teams registered for Category B, and Six teams registered for Category C (Invited). (2) 25 Entries entered Phase-2 Competition on 10 July 2022, including Eleven teams from Category A, Eight teams from Category B, and Six teams from Category C (Invited). (3) 15 Entries entered Phase-3 Competition in Italy and Taiwan on 23 July 2022.
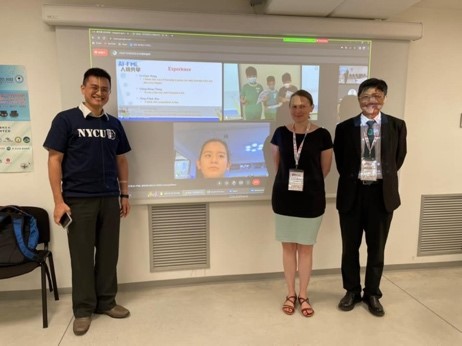 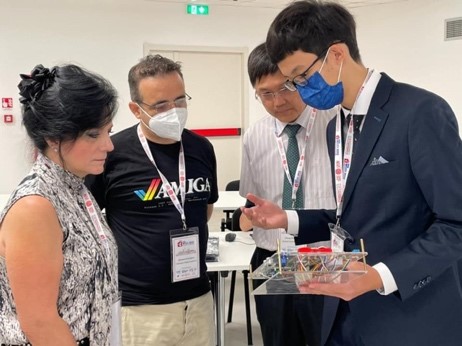 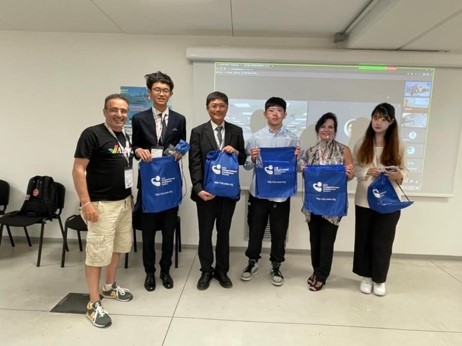
|
|
|
|
IEEE CIS Mentoring Program
In July, at WCCI 2022, the fourth iteration of the IEEE CIS Mentoring Program took place. It was only the second time for it to be run as part of WCCI across all three conferences and research communities—and finally, it was back in person! The program is coordinated by the CIS Mentoring subcommittee and was led by two leads from each of the communities: Nelishia Pillay and Pietro Oliveto for CEC, Amit K. Shukla and Jose Maria Alonso for Fuzz-IEEE, and Benjamin Paassen and Jia Wu for IJCNN. The IJCNN Mentoring was also supported by the International Neural Network Society (INNS) via Zenglin Xu.
The mentoring program itself has grown since its inception, now encompassing one-to-one mentoring for each student and early-career researcher by a senior academic in the field, a dedicated networking event, and an associated Paper Development Workshop (PDW) – see separate article. Prior and throughout the conference, mentees discussed their work with mentors and mentors attended their mentees’ paper presentations, giving feedback and guidance on the subject matter and research in general. At this stage, a big shout and thank you to all the mentors and mentees who went the extra mile to make time for interaction even when regular plans were disrupted by travel problems and Covid. We have had a lot of stories of impromptu Skype and Zoom meetings and plans to follow up contacts made beyond the conference – amazing!
On Tuesday evening, mentees and mentors gathered for the dedicated networking reception which again proved hugely popular, with mentees noting how it facilitated engagement with mentors and fellow mentees, while mentors welcomed the informal setting for discussing with young scholars from across computational intelligence. Some of the really great feedback we have had includes: “A great opportunity to meet between senior and junior researchers”, "I believe it is a very good program with a significant potential of helping young researchers. It was a pleasure to participate for the first time in this program.," "As a student, it is difficult to create new networks, but this program made it easier.," "I think the program is very useful, it helps the mentees to meet people and interact more, not only with senior researcher, but also with each other."
The number of participants was the largest yet, with 70 mentor-mentee pairs and participants from across the world. Following early feedback, the program has been very well received once again, with 88% of participants rating the program, and their interaction with the mentor/mentee very high or high, while 95% of participants would recommend the program to a friend or colleague.
This year’s iteration has been the biggest and we think best-yet, but of course there is always room to improve and grow, especially with some Covid challenges still impacting the participants and program. On a personal note—we started this form of the networking program three years ago, in-person at Fuzz-IEEE 2019 in New Orleans, USA; followed by the formal establishment of the IEEE CIS Mentoring Subcommittee for 2021 to help us build and deliver high-quality mentoring across CIS flagship events. It has been amazing to see the program grow and work with such a dedicated team from across the world. For myself, this will be the last year as chair of the committee, with a great team waiting to take over. I am very much looking forward to passing on the baton and have no doubt that the program will continue to thrive, supporting and contributing to more early—and not-so-early—career research from across our Society.
For now, a big thank you to the whole team, to the WCCI organisers who supported us, to CIS for the generous sponsorship, and above all, to all the participants—keep your eyes peeled for the next iteration of the program soon—and get involved!
Christian Wagner,
IEEE CIS Mentoring SC Chair, on behalf of the IEEE CIS Mentoring Team for WCCI 2022
|
|
|
|
Paper Development Workshop at WCCI 2022
The Paper Development Workshop (PDW) was designed to provide early career authors with guidance and expertise in the development of their manuscript for potential submission to an IEEE CIS journal. The workshop, with a highly-interactive format, includes general presentations of IEEE CIS flagship journals by the Editor-in-Chiefs, as well as roundtable discussions that focus on providing authors with practical and developmental feedback aimed at strengthening and improving their papers.
The PDW started last year at FUZZ-IEEE 2021, unfortunately in a remote way. At WCCI 2022 in Padua, the PDW runed on 18 July by members (Editors-in-Chief and Associate Editors) of the IEEE Transactions on Evolutionary Computation (TEC), IEEE Transactions on Fuzzy Systems (TFS), and IEEE Transactions on Neural Networks and Learning Systems (TNNLS) editorial teams. A big thank you to the Editor-in-Chiefs, Prof. Jonathan Garibaldi (TFS), Prof. Carlos Coello Coello (TEC), that were physically present, and to Prof. Yongduan Song (TMMLS) that unfortunately had to participate remotely.
The PDW had 30 applications from mentees and 20 Associate Editors associated to it. This is quite a remarkable number of participants, considering that the mentees were required to submit a draft journal paper, and that the Associate Editors joined in a completely voluntary way.
The PDW started by an introduction to publishing in IEEE Transactions on Fuzzy Systems, presented by Prof. Jonathan Garibaldi, on behalf of all the flagship journals of IEEE CIS. Prof. Carlos Coello Coello added some important comments, regarding his large experience as Editor-in-Chief of IEEE TEC. A big thank you to both, especially in the Q&A session after the presentations, where a large number of important questions were raised by the early career participants. It was clear that the PDW was highly valuable, especially for PhD students, to hear from the Editor-in Chief of such prestigious journals the dos and don’ts on writing a journal paper.
The program finished with ten small-groups, hands-on sessions between AEs and participants. In general, the groups had three mentees and two associate editors. All groups engaged in fruitful discussions. The mentees received valuable feedback for their future journal publications.
Last but not the least, a big thank you to the whole team (a special thank for Christian Wagner, IEEE CIS Mentoring SC Chair, who had the original idea of running PDW in CIS), to the WCCI organizers and all their supported, to CIS for the sponsorship, and to all the participants that made PDW a remarkable event! We want to make the next PDW an even better event, so get involved and send us your ideas!
João Sousa,
IEEE CIS 2022 Lead of PDW, on behalf of the IEEE CIS Mentoring Team for WCCI 2022
|
|
|
|
When Federated Learning Meets Blockchain: A New Distributed Learning Paradigm
Motivated by the increasingly powerful computing capabilities of end-user equipment, and by the growing privacy concerns over sharing sensitive raw data, a distributed machine learning paradigm known as federated learning (FL) has emerged. By training models locally at each client and aggregating learning models at a central server, FL has the capability to avoid sharing data directly, thereby reducing privacy leakage. However, the conventional FL framework relies heavily on a single central server, and it may fail if such a server behaves maliciously. To address this single point of failure, in this work, a blockchain-assisted decentralized FL framework is investigated, which can prevent malicious clients from poisoning the learning process, and thus provides a self-motivated and reliable learning environment for clients. In this framework, the model aggregation process is fully decentralized and the tasks of training for FL and mining for blockchain are integrated into each participant. Read More
IEEE Computational Intelligence Magazine, August 2022
|
|
|
|
Evolutionary Dual-Ensemble Class Imbalance Learning for Human Activity Recognition
Human activity recognition is an imbalance classification problem in essence since various human actions may occur at different frequencies. Traditional ensemble class imbalance learning methods integrate resampling technique with multi-classifier models, which obtain better generalization than single ones. However, the number of base classifiers is often determined in advance, resulting in the redundant structure of a multi-classifier and larger computational cost. Moreover, various combinations of base classifiers may have similar recognition accuracy, forming a multimodel optimization problem. To address the issue, a dual-ensemble class imbalance learning method is presented, in which two ensemble learning models are nested each other. Read More
Transactions on Emerging Topics in Computational Intelligence, August 2022
|
|
|
|
Concept Drift-Tolerant Transfer Learning in Dynamic Environments
Existing transfer learning methods that focus on problems in stationary environments are not usually applicable to dynamic environments, where concept drift may occur. To the best of our knowledge, the concept drift-tolerant transfer learning (CDTL), whose major challenge is the need to adapt the target model and knowledge of source domains to the changing environments, has yet to be well explored in the literature. This article, therefore, proposes a hybrid ensemble approach to deal with the CDTL problem provided that data in the target domain are generated in a streaming chunk-by-chunk manner from nonstationary environments. At each time step, a class-wise weighted ensemble is presented to adapt the model of target domains to new environments. It assigns a weight vector for each classifier generated from the previous data chunks to allow each class of the current data leveraging historical knowledge independently. Read More
IEEE Transactions on Neural Networks and Learning Systems, August 2022
|
|
|
|
A Brain-Inspired Self-Organizing Episodic Memory Model for a Memory Assistance Robot
This article discusses the implementation of a brain-inspired episodic memory model, which provides memory assistance and tackles the modern public issue of memory impairment embedded as an end-to-end system on the robot companion, Pepper. Based on the fusion adaptive resonance theory, the proposed model can observe and memorize the content of daily events in five aspects: 1) people; 2) activities; 3) times; 4) places; and 5) objects. The model is based on the human memory pipeline, containing a working memory and a two-layer long-term memory model, which can effectively merge, cluster, and summarize past memories based on their context and relevance in a self-organizing manner. When providing memory assistance, the robot can analyze a user query and find the best matching memory cluster to generate verbal cues to stimulate recalling of the target event. Moreover, using reinforcement learning, the robot eventually learns the most effective mapping of cue types to event type through social interaction. Read More
IEEE Transactions on Cognitive and Developmental Systems, June 2022
|
|
|
|
Efficient Reinforcement Learning for StarCraft by Abstract Forward Models and Transfer Learning
Injecting human knowledge is an effective way to accelerate reinforcement learning (RL). However, these methods are underexplored. This article presents our discovery that an abstract forward model [thought-game (TG)] combined with transfer learning is an effective way. We take StarCraft II as our study environment. With the help of a designed TG, the agent can learn a 99% win-rate on a 64×64 map against the Level-7 built-in AI, using only 1.08 h in a single commercial machine. We also show that the TG method is not as restrictive as it was thought to be. It can work with roughly designed TGs, and can also be useful when the environment changes. Comparing with previous model-based RL, we show TG is more effective. We also present a TG hypothesis that gives the influence of different fidelity levels of TG. Read More
IEEE Transactions on Games, June 2022
|
|
|
|
Editor Bing Xue
Victoria University of Wellington, New Zealand Email: [email protected]
|
|
|
|
{{my.Comm Preferences:default=edit me}}
|
|
|
|
|